How I released a hip hop mixtape with MusicLM
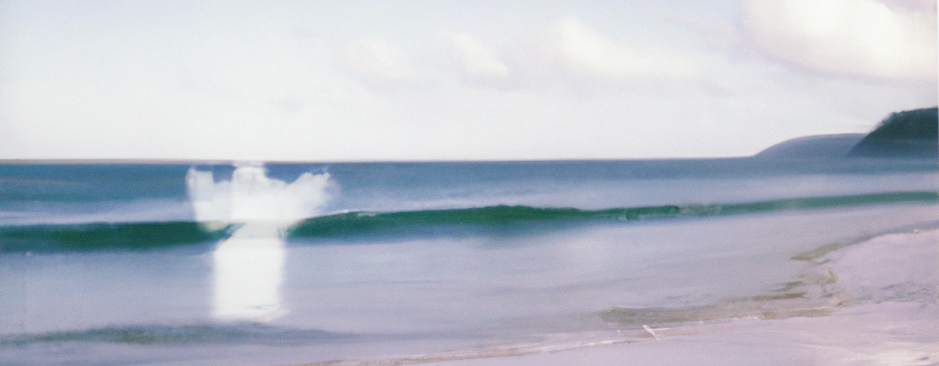
This is a guest post from my friend Ricky Robinett. Ricky and I worked together for nearly a decade on the Developer Network at Twilio, which Ricky led as VP for five years. Today Ricky leads Developer Relations at Cloudflare. In his spare time, Ricky creates hiphop with AI...
Today, I’m releasing a mixtape where all the beats were created with the assistance of generative AI. After creating the beats, I paired them up with pre-existing hip hop vocals (a.k.a. acapellas) to create unofficial remixes.
You can listen to the full version of the mixtape on YouTube.
Generative AI and “Prompt Digging”
When I first got access to Google’s MusicLM, I wondered how I could use it as more than just a novelty. Yes, it was amazing that I could create a short clip of a hardcore punk song out of thin air. But in a practical sense I wouldn’t do anything with this output beyond sending it to a couple friends.
After spending time with MusicLM, I began to think more and more about “crate digging”. As hip hop emerged, being a great DJ wasn’t only about skill but also having the ear to pick out the right records to mix together. And the perseverance to hunt down the rare records no one else could get their hands on. Literally digging through crates of records to find that one record you needed. This whole experience is perhaps best dramatized in the first episode of Netflix’s The Get Down when Shaolin Fantastic is tasked with hunting down a record for Grandmaster Flash.
Quickly, I began to practice what I’d call “prompt digging.” Spending hours with MusicLM trying to find the right prompt to create something I’d want to sample and combine with an acapella to make a full song. Originally MusicLM felt like a toy. But the more time I spent prompt digging, the more it felt like an instrument. Each word of the prompt I could pass to it would shift the output in subtle ways that would bring the output closer, or further, to what I was hoping to find.
For example, my prompts started out simple – like
A early 90’s hip hop beat.
But over time evolved into something that would evoke a more nuanced output – like:
A hip hop beat that you may hear on the radio when you’re hanging out with your friends on a summer night at a playground brooklyn and immediately someone starts freestyling over
or
A catchy hyperpop emo song that you’d hear at a basement party in the midwest in the early 2000s
Experimenting with the subtle shifts that would happen with the sample with each word I added or changed.
The Journey From Sample to Song
I quickly discovered that even though MusicLM was good at generating nuggets of an idea, the output it created wasn’t immediately something I’d call a “song”. There were a few key issues.
The first, and easiest issue to address, was that the output is ~20 seconds long. Of course, loops are something every musician (and developer) is used to. I quickly worked to not only find samples I liked, but samples that would sound good when they were looped.
Second, as I started building the loops, I realized that MusicLM wasn’t always great at staying on beat. Meaning for each clip I’d have to work to make sure each section properly followed the right BPM. I’d also needed to shift the BPM of the sample to match the acapella I thought may fit well with the sample as a remix. This wasn’t a difficult process, but it was a tedious process.
The most difficult problem was that I wasn’t happy with both the overall mix of the output and the way the individual instruments sounded in that mix. MusicLM returns a single audio file that contains all the instruments. I needed a way to treat these instruments as separate tracks like I would when typically creating a song, allowing me to mix each track individually. In trying to solve this problem, I stumbled upon Moises.ai. Moises allowed me to upload the original sample audio created by MusicLM and have it separated by instrument. After this, I’d import the separate files into Logic Pro where I could take a pass at doing a proper mix on the song.
This whole process was very iterative. For each sample I was happy with from MusicLM, I created around 50 samples that I didn’t like. And then, upon breaking apart the tracks with Moises and mixing with Logic Pro only about 1 in every 4 samples were something I could put together a song I liked out of. Meaning both getting a loop I was happy with, and instruments sounding the way I wanted. Personally, the drums and bass were something I was especially critical of. I left a lot on the proverbial cutting room floor because I just couldn’t get the drums or bass to sound right.
Additionally, I wanted to try to produce different styles of music to see where MusicLM excelled and fell short. This meant, many songs didn’t make the final cut because I felt like they were too similar to something else on the mixtape and didn’t showcase the possibilities enough.
To polish things off, I used DALL-E to create an album cover, and ChatGPT to name the songs.
Ultimately, I ended up using what I’m calling the “2600 (MMDC) stack” to create these songs:
MusicLM to generate samples.
Moises.ai to separate a sample into individual audio files for each instrument
DALL-E to generate an album cover
ChatGPT to name the songs
This may, or may not, be the only time this cornucopia of AI tools with a human orchestration layer will be used to create music. But, I’ve learned as a developer that it never hurts to have a fun acronym or nickname for your stack called out from the beginning.
What’s Next?
Something that’s always inspired me about music (and hip hop specifically) is how it embraces innovation and is moved forward by people trying something new – DJ Kool Herc introducing the world to the merry-go-round technique, the Beastie Boys and the Dust Brothers pushing the limits of sampling on Paul’s Boutique, or Girl Talk meticulously mashing up songs in a way no one had ever heard before.
As I was working on this project, I was reading Rick Rubin’s book and this quote really resonated with me:
“All art is a work in progress. It’s helpful to see the piece we’re working on as an experiment. One in which we can’t predict the outcome. Whatever the result, we will receive useful information that will benefit the next experiment. If you start from the position that there is no right or wrong, no good or bad, and creativity is just free play with no rules, it’s easier to submerge yourself joyfully in the process of making things. We’re not playing to win, we’re playing to play. And ultimately, playing is fun. Perfectionism gets in the way of fun.”
― Rick Rubin, The Creative Act: A Way of Being
This certainly isn’t, and won’t be, the best collection of songs created with the assistance of generative AI. It has been a fun experiment, an opportunity to joyfully play and create.
If you’re playing and experimenting with AI, and having fun doing it, please reach out. I’d love to chat, hear more and compare notes.
Find me on X at @rickyrobinett.